Archeologists’ new AI-fueled Northern Star
A new method combining remote sensing and machine learning could have a significant impact on archaeological expeditions conducted in desert settings.
Archeologists’ dreams of thrilling desert expeditions in search of ancient monuments conjure images of Indiana Jones spending months on end in his hat and khaki outfits in the cruel desert heat to find the lost tomb. But what might have taken the likes of real-life Jones months on end to discover in the arid desert conditions can now be found in mere hours using new imaging technologies and artificial intelligence.
A team of researchers from Khalifa University, Sorbonne University Abu Dhabi and Mohamed bin Zayed University of Artificial Intelligence has integrated satellite imaging, namely synthetic aperture radar (SAR), with machine learning (ML) and deep learning (DL) techniques to unearth ancient human settlements and activity buried deep under the UAE’s sands.
The use of these technologies can save several months’ worth of pre-work and guarantee better possibilities for expeditions to successfully locate buried artifacts.
While satellite imaging and radars have previously been used in archeological work, this study, which was published in Geosciences, focuses specifically on the use of these technologies in arid conditions. Arid environments make ground-visual or physical methods difficult to use due to factors like high sand dunes and sandy conditions that interfere with image quality.
“Being able to identify potentially buried artifacts in sand in the environments that we’re exploring is a fairly sophisticated operation,” says Senior Vice President for Research and Development Steven Griffiths, a professor of practice involved in the study. “Our work is focused on this region, which is our differentiator.”
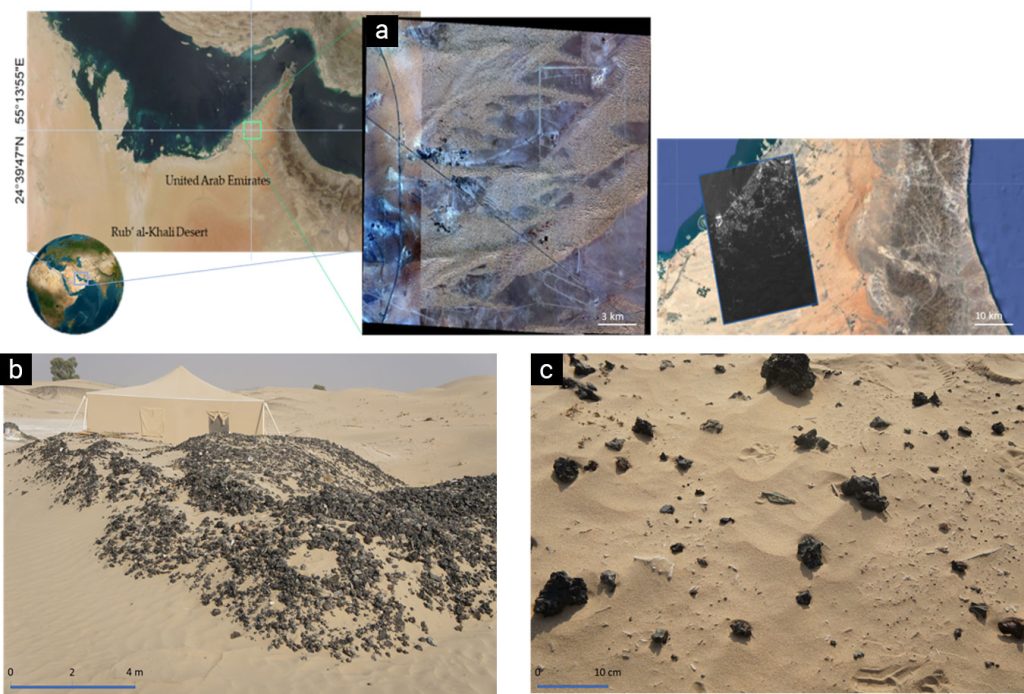
The implications of their research extend beyond the United Arab Emirates to other countries with vast desert areas, including Saudi Arabia, Egypt, Iraq and more. The combined technology could significantly cut the time and resources needed in similar expeditions by automating a lot of the pre-work.
“[The technology] is extraordinarily accessible,” explains Griffiths. “Although the satellite data can be a little expensive, once you have access to the right data from the satellites, and you have people who are competent in machine learning and artificial intelligence, you no longer have to worry about the challenges of financing archaeological expeditions and digging and then finding nothing; we can now screen many potential sites effectively and efficiently. Archeology is not all Indiana Jones style anymore.”
While the technology does have its limitations, frequent use can address these challenges. Machine learning algorithms may initially struggle to differentiate between different surface materials or archaeological features, modeling and prediction accuracies can improve using the neural network of backpropagation algorithms based on the performed cluster groups following recent field surveys.
“It is really neat to see how much potential is here that has not yet been uncovered,” Griffiths concludes. “We’re excited about that.”
Reference
- Ben-Romdhane, H. et al. Detecting and Predicting Archaeological Sites Using Remote Sensing and Machine Learning—Application to the Saruq Al-Hadid Site, Dubai, UAE. Geosciences 13(6), 179 (2023). | Article